Google Search To Show If An Image Is AI Generated, Edited Or Taken With Camera.
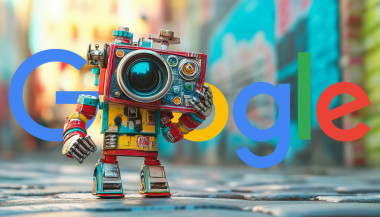
There have been multiple accounts created with the sole purpose of posting advertisement posts or replies containing unsolicited advertising.
Accounts which solely post advertisements, or persistently post them may be terminated.